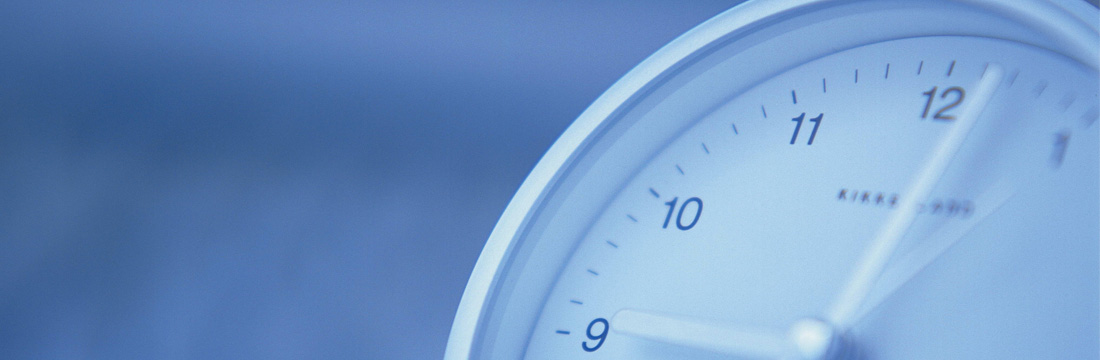
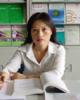
王文剑
计算智能、数据挖掘、神经网络、支撑向量机、环境计算等。
个性化签名
- 姓名:王文剑
- 目前身份:
- 担任导师情况:
- 学位:
-
学术头衔:
博士生导师, 教育部“新世纪优秀人才支持计划”入选者
- 职称:-
-
学科领域:
计算机科学技术
- 研究兴趣:计算智能、数据挖掘、神经网络、支撑向量机、环境计算等。
王文剑,女,1968年10月出生,博士,教授,博士生导师,山西大学计算机与信息技术学院副院长,入选教育部新世纪优秀人才支持计划、山西省青年学术带头人,山西省青年科研专家、太原市科技明星。山西大学计算智能与中文信息处理省部共建教育部重点实验室副主任,山西大学智能信息处理山西省重点实验室副主任,山西省高等学校优秀科研创新团队主要成员。先后在国内外重要学术刊物上和重要国际会议上发表论文70余篇,其中被sci/ei检索40余篇,被同行他引180余次。
主要研究方向为:计算智能、数据挖掘、神经网络、支撑向量机、环境计算等。
-
凯发k8国际首页主页访问
1878
-
关注数
0
-
成果阅读
1275
-
成果数
20
上传时间
2010-03-30
王文剑, 郭虎升
计算机工程与应用,2009,45(2):51~54,-0001,():
-1年11月30日
针对支持向量机(suppon vector machine,svm)对大规模样本分类效率低下的问题,提出了基于自适应共振理论(adaptive resonance theory,art)神经网络与自组织特征映射(self-organizingfeature map,som)神经网络的svm训练算法,分别称为art-svm算法与som-svm算法。这两种算法通过聚类压缩数据集,使svm训练的速度大大提高,同时可获得令人满意的泛化能力。
支持向量机, art-svm算法, som-svm算法, 聚类
-
111浏览
-
0点赞
-
0收藏
-
0分享
-
354下载
-
引用
上传时间
2010-03-30
王文剑, wen-jian wang
proceedings of the seventh international conference on machine learning and cybernetics, kunming, 12-15 july 2008,-0001,():
-1年11月30日
this paper presents an improved incremental learning technique for svm, namely redundant incremental svm (risvm), for pattern classification problems. through adding some non-support vectors (say, redundant vectors in the sense of contribution to the final solution) at each incremental step, the risvm algorithm can achieve similar performance to the svm in batch (or non-incremental svm) but result in less support vectors for the same quality of pattern classification, and also it can provide better generalization performance in comparison with other incremental techniques for svm. the bispiral problem and five widely used benchmark data sets are employed to verify the method, and the simulations support the feasibility and effectiveness of the proposed approach.
classification, incremental learning, redundant vector, support vector machine
-
81浏览
-
0点赞
-
0收藏
-
0分享
-
114下载
-
引用
上传时间
2010-03-30
王文剑, 张妤, 康向平
计算机科学,2008,35(4):178~180,-0001,():
-1年11月30日
泛化能力是机器学习关心的一个根本问题,采用集成学习技术可以有效地提高泛化能力。本文提出了一种将支持向量机(support vector machine,svm)进行选择性集成回归的方法。通过引入三个阈值,可以选择合适的子svm,从而进一步提高了整个集成学习的效率。实验结果表明,本文提出的选择性集成方法可以在一定程度上解决svm的模型选择问题和大规模数据集的学习问题,与传统的集成方法bagging相比具有更高的泛化能力。
支持向量机,, 集成学习,, 回归,, bagging
-
113浏览
-
0点赞
-
0收藏
-
0分享
-
223下载
-
引用
上传时间
2010-03-30
王文剑, 侯岩
计算机科学,2008,35(3):49~51,-0001,():
-1年11月30日
随着电子邮件的广泛应用,泛滥成灾的垃圾邮件对人们的生活和网络安全带来了严重的威胁,反垃圾邮件问题已成为全球性的具有现实意义的问题。本文提出了一种基于动态特征词典的svm中文邮件过滤方法,通过动态构造特征词典以及选择合适的支持向量机(support vector machine,svm)核参数,有效地提高了垃圾邮件的过滤精度,实验结果超过了网易免费邮所公布的过滤指标。
支持向量机,, 中文电子邮件,, 过滤,, 动态特征词典
-
64浏览
-
0点赞
-
0收藏
-
0分享
-
109下载
-
引用
上传时间
2010-03-30
王文剑, 门昌骞
广西师范大学学报(自然科学版),2008,26(1):186~189,-0001,():
-1年11月30日
传统的支撑向量机是一种有监督学习方法,为了提高其泛化能力,提出了一种新的基于多学习器标记的半监督svm学习方法。该方法将训练集分为有标记样本和无标记样本两个集合,通过对无标记样本进行分别标记,不断修正有标记样本集规模,从而提高svm的泛化性能。在中文电子邮件过滤数据集上的实验结果证明了该方法的可行性和有效性。
半监督学习, 支持向量机, 邮件过滤
-
97浏览
-
0点赞
-
0收藏
-
0分享
-
351下载
-
引用
上传时间
2010-03-30
王文剑, wenjian wanga, *, changqian mena, weizhen lub
neurocomputing 71(2008)550-558,-0001,():
-1年11月30日
for time-series forecasting problems, there have been several prediction models to data, but the development of a more accurate model is very difficult because of high non-linear and non-stable relations between input and output data. almost all the models at hand are not applicable online, although online prediction, especially for air quality parameters forecasting, has very important significance for realworld applications. a support vector machine (svm), as a novel and powerful machine learning tool, can be used for time-series prediction and has been reported to perform well by some promising results. this paper develops an online svm model to predict air pollutant levels in an advancing time-series based on the monitored air pollutant database in hong kong downtown area. the experimental comparison between the online svm model and the conventional svm model (non-online svm model) demonstrates the effectiveness and efficiency in predicting air quality parameters with different time series.
airpollutant, online model, prediction performance, support vector machine, time-series forecasting
-
107浏览
-
0点赞
-
0收藏
-
0分享
-
105下载
-
引用
上传时间
2010-03-30
王文剑, 王平
计算机工程与设计,2008,29(4):901~903,-0001,():
-1年11月30日
不变性常识与支持向量机的融合技术是近年来支持向量机研究的重点之一,将不变性常识融合于学习模型,有助于提高模型的泛化能力。提出了一种新的不变性常识与支持向量机的融合方法,该方法通过最佳逼近点来代表不变性变换形成的轨迹簇将不变性常识融合于svm。将该方法应用于mnist手写数字数据库,与经典svm方法及virtualsv(vsv)方法的对比实验结果表明,该方法可以提高svm的泛化能力。
支持向量机, 不变性常识, 最佳逼近点, 融合方法
-
66浏览
-
0点赞
-
0收藏
-
1分享
-
70下载
-
引用
上传时间
2010-03-30
王文剑, 郭金玲
电脑开发与应用,2007,20(12):50~51,-0001,():
-1年11月30日
选择是支撑向量机(support vector machine,svm)研究中的核心问题之一。提出了一种基于数据分布特征的svm核函数及其参数选择的方法。首先分析了确定数据分布特征的重要性,然后给出了判断数据呈高斯分布的方法,并探讨了svm核函数及其参数选择与数据分布的相关性。数值实验说明了本文提出的方法的可行性与有效性。
支撑向量机,, 核参数选择,, 高斯分布
-
59浏览
-
0点赞
-
0收藏
-
0分享
-
106下载
-
引用
上传时间
2010-03-30
王文剑, 马亮
广西师范大学学报(自然科学版),2007,25(4):59~62,-0001,():
-1年11月30日
提出一种选择支持向量分类(support vector classification,svc)最优核参数的算法,称为mi(maximum isolation)算法,通过定义样本间的独立性,可以获得最优核参数和相应的最优学习模型。该算法可以在支持向量机训练之前得到最优的核参数,计算代价较小,实验证明所提出的算法简单有效。
支持向量机, 核参数, mi算法, 数据独立性
-
63浏览
-
0点赞
-
0收藏
-
1分享
-
72下载
-
引用
上传时间
2010-03-30
王文剑, 侯岩
山西大学学报(自然科学版),2007,30(3):303~309,-0001,():
-1年11月30日
提出了一种基于svm的中文邮件过滤方法,通过构造最优的支持向量机(support vector machine,svm)过滤模型,有效地提高了垃圾邮件的过滤精度,实验结果超过了网易免费邮所公布的过滤指标。
支持向量机, 中文电子邮件, 过滤, 模型选择
-
67浏览
-
0点赞
-
0收藏
-
0分享
-
84下载
-
引用